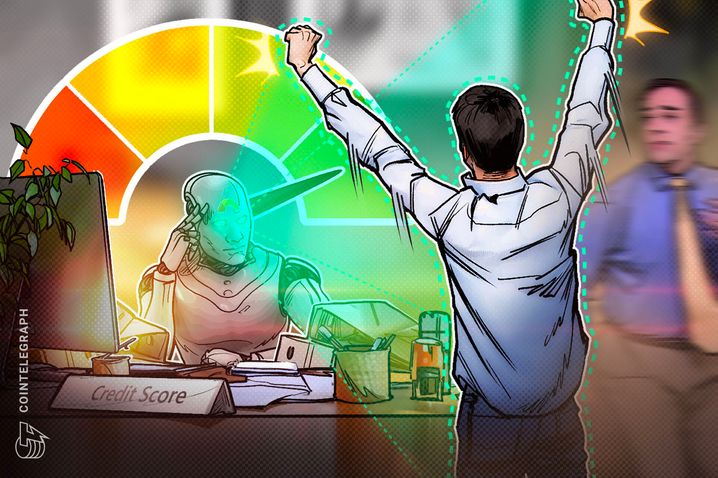
What is AI-based credit scoring?
AI-based credit scoring is a modern approach to assessing an individual’s creditworthiness that involves the use of artificial intelligence (AI) and machine learning (ML) technologies.
Instead of solely relying on traditional methods of credit evaluation, such as the review of credit history, income and existing debts, AI-based credit scoring considers a broader range of data sources, like digital footprints.
AI-based credit scoring uses complex algorithms to analyze this data and predict an individual’s future financial behavior. This advanced form of credit scoring has the potential to provide more nuanced insights and help lenders make more informed decisions.
The use of AI in credit scoring also opens the door to assessing the creditworthiness of individuals who may not have a traditional credit history but leave other kinds of data footprints that can be evaluated, such as online transactions, social media interactions, browsing behaviors or mobile app usage.
How does AI-based credit scoring work?
The functioning of AI-based credit scoring is rooted in machine learning algorithms. These algorithms are trained on large sets of historical data, from which they identify patterns and correlations related to a borrower’s ability or likelihood to repay a loan.
The patterns learned from past data are then applied to new data to predict future behavior. This process of learning from past data to make future predictions is a key characteristic of machine learning, and it’s what makes AI-based credit scoring possible.
The data utilized in AI-based credit scoring can come from numerous sources. Traditional credit information, including payment history, existing debts, and length of credit history, are common data sources. However, AI-based credit scoring also incorporates alternative data sources, giving it a potential edge over traditional credit scoring methods. These alternative data sources can include transaction data, users’ internet browsing behavior, social media activity and other forms of digital footprints.
By analyzing a broad range of data, AI-based credit scoring models can potentially offer a more comprehensive and accurate assessment of credit risk. They provide a holistic view of a person’s financial behavior, including aspects that traditional credit scoring methods might overlook.
Types of AI-based credit scoring models
AI-based credit scoring models can come in various types, depending mainly on the specific machine learning techniques and data sources used. These types include supervised learning models, unsupervised learning models and hybrid models.
Supervised learning models
Supervised learning models are common in AI-based credit scoring. They are trained on a labeled data set with known outcomes, such as credit history and loan repayment behavior. The model learns to associate input data with output data and predicts the creditworthiness of new individuals based on their credit history.
Unsupervised learning models
Unsupervised learning models are used when outcomes are unknown. They are trained on unlabeled data sets, discovering patterns within the data. In credit scoring, an unsupervised model may cluster individuals based on transaction data or browsing behavior. These clusters can infer individuals’ creditworthiness.
Advertisement
Stay safe in Web3. Learn more about Web3 Antivirus →
Hybrid learning models
Hybrid models combine both supervised and unsupervised learning techniques. They use unsupervised learning to discover new data features or relationships, and supervised learning for predictions. Due to their flexibility and adaptability, hybrid models are well-suited for credit scoring, leveraging the strengths of both learning types for comprehensive credit risk assessment.
Traditional credit scoring vs. AI-based credit scoring
Traditional and AI-based credit scoring represent two distinct approaches to evaluating an individual’s creditworthiness. Traditional credit scoring systems typically use a relatively small set of variables, such as payment history, the length of credit history, the amount of outstanding debt and the types of credit in use. However, such systems may also exclude people who lack a traditional credit history, such as young adults or individuals in developing countries.
AI-based credit scoring, on the other hand, uses machine learning algorithms to analyze a vast array of data, going beyond traditional credit information.
Risks of AI-based credit scoring
While AI-based credit scoring brings innovation and the potential for improved accuracy in assessing creditworthiness, it also introduces certain risks that need to be managed.
Transparency and explainability
Due to the complexity of AI models, understanding the logic behind a certain credit score can be challenging. This “black box” problem can complicate regulatory compliance, especially in places where lenders must explain credit decisions to consumers.
Potential for bias and discrimination
AI models may perpetuate biases if the training data is skewed or unrepresentative. This can lead to unfair credit decisions affecting specific individuals or groups.
Privacy and data security concerns
Using non-traditional data sources like social media or browsing behavior introduces privacy and data security risks. Misusing personal data or data security breaches can harm individuals and tarnish lenders’ reputations.
Data quality
AI-based credit scoring models heavily depend on the training data’s accuracy, completeness and freshness. Poor quality data can result in inaccurate predictions and potentially unfair credit decisions.
Risk management and regulatory guidelines
It is crucial for lenders to effectively manage these risks, and for regulatory bodies to establish clear guidelines to ensure the responsible use of AI-based credit scoring technologies.
Can AI-based credit scoring and blockchain technology be amalgamated?
Integrating AI-based credit scoring and blockchain technology is possible, and it could lead to more reliable and secure systems for credit assessment. Blockchain technology — renowned for its unalterable and secure characteristics — can significantly increase the transparency and security of the data that feeds into AI-based credit scoring systems.
Integrating blockchain technology could allow for a decentralized and immutable record of credit-related transactions. Once recorded on the blockchain, each transaction cannot be altered or deleted, providing a reliable data source for AI algorithms.
This can increase the trustworthiness of the data used for training AI models, leading to more reliable credit scores. Moreover, blockchain’s transparent nature could also help address the “black box” problem associated with AI-based credit scoring, as it could offer a clear and auditable trail of how the data has been used and processed.
However, such integration also introduces new challenges. For instance, implementing blockchain technology can be complex and costly, and there are also potential privacy concerns due to the transparent nature of blockchain transactions.
Furthermore, while blockchain can improve the transparency and integrity of data, it doesn’t automatically resolve data bias or representativeness issues. As such, careful thought and planning are needed to successfully combine these two technologies to maximize their strengths and mitigate potential risks.
The future of AI-based credit scoring
The future of AI-based credit scoring is likely to be marked by continuous advancements and increased adoption across the financial sector. As machine learning algorithms become more sophisticated and robust, and alternative data sources become more readily available, AI-based credit scoring models are expected to become even more accurate and comprehensive in assessing credit risk.
The potential to provide a more inclusive credit evaluation system — one that considers individuals who lack a traditional credit history — will be a significant driving force for the continued development and uptake of these models.
However, the future will also bring challenges that need to be addressed. Questions of transparency, bias, and data privacy and security will remain paramount as these models become more prevalent.
Ensuring that AI-based credit scoring is used responsibly and ethically requires ongoing efforts from industry and regulatory bodies. Similarly, advances in related technologies, such as blockchain, may provide opportunities to enhance the transparency and security of AI-based credit scoring, but will also bring their own complexities